Robot communication: It's more than just talk
As robots start to spill out of factories and into more human spaces, researchers try to better equip both parties to understand each other.
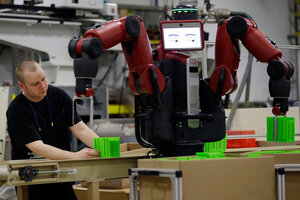
A technician works with Baxter, an adaptive manufacturing robot created by Rethink Robotics, at The Rodon Group manufacturing facility, in Hatfield, Pa., March 12, 2013.
Matt Rourke/AP
C-3PO’s fluency in more than 6 million forms of communication in “Star Wars” set a high bar for human-robot interaction, and the field has been struggling to catch up ever since.
They started in the factories, taking over physically demanding and repetitive tasks. Now robots are moving into hospitals, shopping malls, even the International Space Station, and experts don’t expect their expansion into human spaces to slow down anytime soon.
“Even 10 years ago, the primary use of the robots was in the dangerous, dirty, and dull work,” says Julie Shah, an engineering professor at the Massachusetts Institute of Technology in Cambridge, Mass. “You’d deploy them to operate remotely from people, but [now] robots are integrating into all aspects of our lives relatively quickly.”
Freed from their isolated industrial cages, robots navigating the human world can pose hazards to themselves and others, so researchers are seeking ways to prepare for a future where people and robots can work safely together.
While they wouldn’t have made his official list, C3PO’s most important forms of communication may have been nonverbal. We absorb a staggering amount of information visually, from gestures and facial expressions to traffic lights and turn signals, and good design can take advantage of that skill to let humans meet robots halfway.
Signaling to others
Holly Yanco, computer science professor at the University of Massachusetts, Lowell, suggests early measures could be as simple as equipping robots with universal icons.
“I may not need to know everything that the robot is doing, but I need to know that this space is safe for me to walk into,” explains Professor Yanco.
A survey conducted by her graduate student, Daniel Brooks, found traffic light colors overlaid with simple symbols such as checks or question marks sufficient to communicate a robot’s status to untrained bystanders. Think of the “Halt!” robots from “Wall-E.”
Such iconography still depends on culture, Yanco is quick to point out. Another path involves giving robots something all humans have experience reading.
Rethink Robotics takes this approach with its dual-armed Baxter, which features a cartoon face displayed on a swiveling tablet. Thanks to Baxter’s animated eyes, human coworkers can know at a glance where its attention lies and which arm it may be moving next.
People watching
Even if robots become open books, that’s only half of the equation. Dr. Shah heads MIT’s Interactive Robotics Group, a lab focused on giving robots mental and sensory flexibility to complement their physical prowess.
They aim to build robotic systems that can work alongside, and even integrate with, human teams. And that means robots that learn from observation, predict teammate actions, and adjust their behavior accordingly, much like a person would. “I don’t think this is a very futuristic idea anymore,” Shah says.
In fact, the group tested just such a system last year. After an “apprenticeship” spent watching nurses and doctors, a robotic decision support system succeeded in making patient care suggestions that nurse participants in controlled experiments accepted 90 percent of the time. The study culminated in a pilot deployment on the labor and delivery floor of a Boston hospital, where the system gathered inpatient data from handwriting on a whiteboard and offered real time advice.
“That was the first time anybody has been able to demonstrate a system learning so efficiently with so few demonstrations in a real world setting,” says Shah. “It can be done.”
Still, even the most mentally dextrous teammate will sink a project if they can’t keep out of the way. “When you start working in a confined space, an elbow-to-elbow space, body posture and motion signals become very important. People rely on them,” says Shah.
Her team also harnesses machine learning and biophysical modeling to help robots read human body language, and predict where a teammate will move next. For example, tracking a person’s walking speed and head direction reveals which way they’ll turn about two steps early, information we humans only become aware of when a miscalculation ends in the “hallway dance.”
“Clearly we all use these cues everyday but we don’t think about it,” says Shah. “Just a quarter of a second or half a second of an arm reaching movement ... with just the slightest motion of their elbow or their shoulder, we can train a machine learning technique to predict with 75 percent accuracy where they’re going to reach.”
Reading minds?
While Yanco and Shah help catch robots up to people’s signaling and interpreting abilities, other researchers see no reason to limit robots to human senses. Another system developed by MIT’s Computer Science and Artificial Intelligence Laboratory (CSAIL) can read minds. Or at least one very specific thought.
While researching brain-to-computer interfaces with monkeys, Boston University Prof. Frank Guenther was struck by how one particular signal came through unusually clearly against the cacophonous background of neural activity. When the monkeys noticed that the computer under their control had made a mistake, a cursor moving right when they had thought “go left,” for example, the system registered a so-called “error potential.”
The signal was strong enough to be detected via an electrical cap and featured a relatively similar shape from person to person. A collaboration between CSAIL and Dr. Guenther’s lab succeeded in designing a system that let a Baxter robot sort paint cans and wire spools into two buckets by “listening” for error potentials, randomly guessing at first and then self-correcting if it noticed the user thinking it made a mistake.
At around 85 percent accuracy, the system isn’t ready for the factory floor, but Guenther expects eventual applications such as a human overseeing a self-driving car or a supervisor monitoring manufacturing machines.
“We’re capitalizing on the fact that the human brain already has a tremendous amount of circuitry built for understanding things and if it sees a mistake, that mistake can be at a pretty high level and still generate a signal,” he says.
And there’s no reason to expect machines to stop at error potentials. Guenther can imagine a future where smartphone cameras measure pupil dilation and cases measure skin resistance (much like today’s lie detectors) to read the user’s emotions and respond more empathically.
A functional C-3PO may still be a long way off, but Yanco agrees that we’ve just begun to see what’s possible when robots and humans join forces. “We’re still in the very early days,” says Yanco. “I think there’s still a lot of exploration to go.”